Lately, there’s been a surge of instruments claiming to detect AI-generated content material with spectacular accuracy. However can they actually do what they promise? Let’s discover out! A latest tweet by Christopher Penn exposes a serious flaw: an AI detector confidently declared that the US Declaration of Independence was 97% AI-generated. Sure, a doc written over 240 years in the past, lengthy earlier than synthetic intelligence existed, was flagged as principally AI-generated.
This case highlights a vital situation: AI content material detectors are unreliable and infrequently outright incorrect. Regardless of their claims, these instruments depend on simplistic metrics and flawed logic, resulting in deceptive outcomes. So, earlier than you belief an AI detector’s verdict, it’s value understanding why these instruments could be extra smoke than substance.
Notably, Wikipedia, an vital supply of coaching information for AIs, noticed not less than 5% of latest articles in August being AI-generated. In an analogous context, I discovered a latest examine by Creston Brooks, Samuel Eggert, and Denis Peskoff from Princeton College, titled The Rise of AI-Generated Content material in Wikipedia, sheds mild on this situation. Their analysis explores the implications of AI-generated content material and assesses the effectiveness of AI detection instruments like GPTZero and Binoculars.
This text will summarise the important thing findings, analyse the effectiveness of AI detectors, and talk about the moral concerns surrounding their use, particularly in tutorial settings.
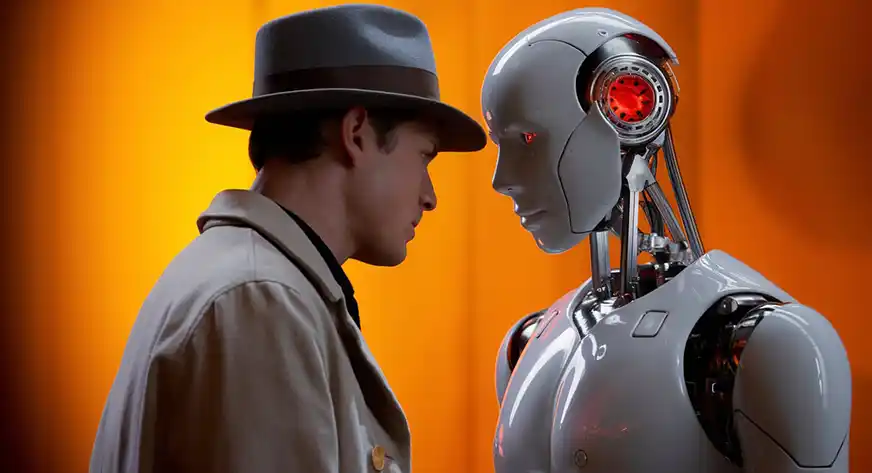
The Rise of AI-Generated Content material in Wikipedia
Synthetic Intelligence (AI) has grow to be a double-edged sword within the digital age, providing each exceptional advantages and severe challenges. One of many rising issues is the proliferation of AI-generated content material on widely-used platforms reminiscent of Wikipedia.
AI Content material Detection in Wikipedia
The examine centered on detecting AI-generated content material throughout new Wikipedia articles, notably these created in August 2024. Researchers used two detection instruments, GPTZero (a business AI detector) and Binoculars (an open-source different), to analyse content material from English, German, French, and Italian Wikipedia pages. Listed below are some key factors from their findings:
- Improve in AI-Generated Content material:
- The examine discovered that roughly 5% of newly created English Wikipedia articles in August 2024 contained vital AI-generated content material. This marked a noticeable enhance in comparison with pre-GPT-3.5 releases (earlier than March 2022), the place the brink was calibrated to a 1% false optimistic price.
- Decrease percentages had been noticed for different languages, however the pattern was constant throughout German, French, and Italian Wikipedia.
- Traits of AI-Generated Articles:
- Articles flagged as AI-generated had been typically of decrease high quality. That they had fewer references, had been much less built-in into Wikipedia’s broader community, and generally exhibited biased or self-promotional content material.
- Particular traits included self-promotion (e.g., articles created to advertise companies or people) and polarizing political content material, the place AI was used to current one-sided views on controversial subjects.
- Challenges in Detecting AI-Generated Content material:
- Whereas AI detectors can determine patterns suggestive of AI writing, they face limitations, notably when the content material is a mix of human and machine enter or when articles endure vital edits.
- False positives stay a priority, as even well-calibrated techniques can misclassify content material, complicating the evaluation course of.
Evaluation of AI Detectors: Effectiveness and Limitations
The analysis reveals vital insights into the efficiency and limitations of AI detectors:
- Efficiency Metrics:
- Each GPTZero and Binoculars aimed for a 1% false optimistic price (FPR) on a pre-GPT-3.5 dataset. Nevertheless, over 5% of latest English articles had been flagged as AI-generated regardless of this calibration.
- GPTZero and Binoculars had overlaps but additionally confirmed tool-specific inconsistencies, suggesting that every detector has its personal biases and limitations. For instance, Binoculars recognized extra AI-generated content material in Italian Wikipedia in comparison with GPTZero, seemingly on account of variations of their underlying fashions.
- Black-Field vs. Open-Supply:
- GPTZero operates as a black-box system, which means customers have restricted perception into how the software makes its choices. This lack of transparency could be problematic, particularly when coping with nuanced instances.
- Binoculars, however, is open-source, permitting for higher scrutiny and adaptableness. It makes use of metrics like cross-perplexity to find out the probability of AI involvement, providing a extra clear strategy.
- False Positives and Actual-World Influence:
- Regardless of efforts to reduce FPR, false positives stay a vital situation. An AI detector’s mistake can result in wrongly flagging legit content material, doubtlessly eroding belief within the platform or misinforming readers.
- Moreover, the usage of detectors in non-English content material confirmed various charges of accuracy, indicating a necessity for extra strong multilingual capabilities.
Moral Concerns: The Morality of Utilizing AI Detectors
AI detection instruments have gotten more and more widespread in academic establishments, the place they’re used to flag potential instances of educational dishonesty. Nevertheless, this raises vital moral issues:
- Inaccurate Accusations and Scholar Welfare:
- It’s morally incorrect to make use of AI detectors in the event that they produce false positives that unfairly accuse college students of dishonest. Such accusations can have severe penalties, together with tutorial penalties, broken reputations, and emotional misery.
- When AI detectors wrongly flag college students, they face an uphill battle to show their innocence. This course of could be unfair and stigmatizing, particularly when the AI software lacks transparency.
- Scale of Use and Implications:
- In line with latest surveys, about two-thirds of academics repeatedly use AI detection instruments. At this scale, even a small error price can result in lots of or hundreds of wrongful accusations. The impression on college students’ academic expertise and psychological well being can’t be understated.
- Academic establishments must weigh the dangers of false positives in opposition to the advantages of AI detection. They need to additionally think about extra dependable strategies of verifying content material originality, reminiscent of process-oriented assessments or reviewing drafts and revisions.
- Transparency and Accountability:
- The analysis highlighted the necessity for higher transparency in how AI detectors operate. If establishments depend on these instruments, they have to clearly perceive how they work, their limitations, and their error charges.
- Till AI detectors can provide extra dependable and explainable outcomes, their use must be restricted, notably when a false optimistic might unjustly hurt a person’s fame or tutorial standing.
The Influence of AI-Generated Content material on AI Coaching Information
As AI fashions develop in sophistication, they eat huge quantities of information to enhance accuracy, perceive context, and ship related responses. Nevertheless, the rising prevalence of AI-generated content material, particularly on distinguished knowledge-sharing platforms like Wikipedia, introduces complexities that may affect the standard and reliability of AI coaching information. Right here’s how:
Threat of Mannequin Collapse by way of Self-Referential Information
With the expansion of AI-generated content material on-line, there’s a rising concern that new AI fashions could find yourself “coaching on themselves” by consuming datasets that embrace giant parts of AI-produced info. This recursive coaching loop, also known as “mannequin collapse,” can have severe repercussions. If future AI fashions rely too closely on AI-generated information, they danger inheriting and amplifying errors, biases, or inaccuracies current in that content material. This cycle might result in the degradation of the mannequin’s high quality, because it turns into more durable to discern factual, high-quality human-generated content material from AI-produced materials.
Reducing the Quantity of Human-Created Content material
The fast enlargement of AI in content material creation could cut back the relative quantity of human-authored content material, which is vital for grounding fashions in genuine, well-rounded views. Human-generated content material brings distinctive viewpoints, delicate nuances, and cultural contexts that AI-generated content material typically lacks on account of its dependence on patterns and statistical chances. Over time, if fashions more and more prepare on AI-generated content material, there’s a danger that they could miss out on the wealthy, various info offered by human-authored work. This might restrict their understanding and cut back their functionality to generate insightful, unique responses.
Elevated Potential for Misinformation and Bias
AI-generated content material on platforms like Wikipedia has proven traits towards polarizing or biased info, as famous within the examine by Brooks, Eggert, and Peskoff. AI fashions could inadvertently undertake and perpetuate these biases, spreading one-sided or misguided views if such content material turns into a considerable portion of coaching information. For instance, if AI-generated articles continuously favour explicit viewpoints or omit key particulars in politically delicate subjects, this might skew the mannequin’s understanding and compromise its objectivity. This turns into particularly problematic in healthcare, finance, or regulation, the place bias and misinformation might have tangible unfavourable impacts.
Challenges in Verifying Content material High quality
Not like human-generated information, AI-produced content material can generally lack rigorous fact-checking or exhibit a formulaic construction that prioritizes readability over accuracy. AI fashions skilled on AI-generated information could study to prioritize these identical qualities, producing content material that “sounds proper” however lacks substantiated accuracy. Detecting and filtering such content material to make sure high-quality, dependable information turns into more and more difficult as AI-generated content material turns into extra refined. This might result in a gradual degradation within the trustworthiness of AI responses over time.
High quality Management for Sustainable AI Growth
AI fashions want a coaching course of for sustainable progress that maintains high quality and authenticity. Like these mentioned within the analysis, content material verification techniques will play a vital function in distinguishing between dependable human-authored information and doubtlessly flawed AI-generated information. Nevertheless, as seen with the instance of false positives in AI detection instruments, there’s nonetheless a lot to enhance earlier than these techniques can reliably determine high-quality coaching information. Hanging a stability the place AI-generated content material dietary supplements fairly than dilutes coaching information might assist keep mannequin integrity with out sacrificing high quality.
Implications for Lengthy-Time period Information Creation
AI-generated content material has the potential to broaden data, filling gaps in underrepresented subjects and languages. Nevertheless, this raises questions on data possession and originality. If AI begins to drive the majority of on-line data creation, future AI fashions could grow to be extra self-referential, missing publicity to various human concepts and discoveries. This might stifle data, as fashions replicate and recycle comparable content material as an alternative of evolving with new human insights.
AI-generated content material presents each a possibility and a danger for coaching information integrity. Whereas AI-created info can broaden data and enhance accessibility, vigilant oversight is required to make sure that recursive coaching doesn’t compromise mannequin high quality or propagate misinformation.
Conclusion
The surge of AI-generated content material is a transformative drive with promise and perils. It introduces environment friendly content material creation whereas elevating dangers of bias, misinformation, and moral complexities. Analysis by Brooks, Eggert, and Peskoff reveals that though AI detectors, reminiscent of GPTZero and Binoculars, can flag AI content material, they’re nonetheless removed from infallible. Excessive false-positive charges pose a selected concern in delicate environments like training, the place an inaccurate flag might result in unwarranted accusations with severe penalties for college students.
A further concern lies within the potential results of AI-generated content material on future AI coaching information. As platforms like Wikipedia accumulate AI-generated materials, there’s an rising danger of “mannequin collapse,” the place future AI fashions are skilled on partially or closely AI-produced information. This recursive loop might diminish mannequin high quality, as AI techniques could amplify inaccuracies or biases embedded in AI-generated content material. Relying too closely on AI-produced information might additionally restrict the richness of human-authored views, lowering fashions’ capability to seize nuanced, various viewpoints important for high-quality output.
Given these limitations, AI detectors shouldn’t be seen as definitive gatekeepers of authenticity however as instruments to enhance a multi-faceted strategy to content material analysis. Over-reliance on AI detection alone—particularly when it might yield flawed or deceptive outcomes—could be insufficient and doubtlessly damaging. Establishments, subsequently, should rigorously stability integrating AI detection instruments with broader, extra nuanced verification strategies to uphold content material integrity whereas prioritizing equity and transparency. In doing so, we will embrace the advantages of AI in data creation with out compromising on high quality, authenticity, or moral requirements.
In case you are on the lookout for a Generative AI course on-line, then discover: GenAI Pinnacle Program
Steadily Requested Questions
Ans. AI detectors are sometimes unreliable, continuously producing false positives and flagging human-written content material as AI-generated.
Ans. This incident highlights flaws in AI detectors, which generally depend on oversimplified metrics that result in incorrect assessments.
Ans. AI-generated content material can introduce biases and misinformation and should complicate high quality management for future AI coaching information.
Ans. False positives from AI detectors can wrongly accuse college students of dishonest, resulting in unfair tutorial penalties and emotional misery.
Ans. There’s a danger of “mannequin collapse,” the place AI fashions prepare on AI-generated information, doubtlessly amplifying inaccuracies and biases in future outputs.