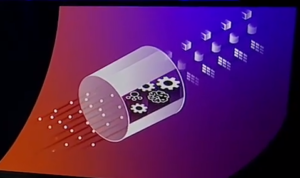
AWS SageMaker has lengthy served because the go-to platform for managing all the lifecycle of machine studying (ML) and GenAI fashions. It gives instruments to construct, practice, and deploy these fashions. The platform can also be used to entry pre-trained fashions, construct basis fashions (FMs), and refine datasets.
Nevertheless, there was a rising want for extra instruments to deal with different facets of the ML lifecycle, resembling governance instruments and automatic validation. Whereas varied instruments exist to handle such wants, lots of them function exterior the SageMaker ecosystem. This fragmentation usually provides complexity, inefficiency, and elevated overhead for customers.
To handle these challenges, AWS has launched a complete setting with its next-generation SageMaker options, introduced on the re:Invent 2024 convention. The replace is designed to supply a unified hub for knowledge, analytics, and AI instruments.
The introduction of the next-generation SageMaker comes at a time when there’s a rising pattern of enterprises utilizing knowledge in interconnected methods. This convergence of AI and analytics may assist allow companies to leverage their knowledge for a variety of capabilities, resembling enhancing predictive upkeep and enhancing buyer personalization.
“We’re seeing a convergence of analytics and AI, with clients utilizing knowledge in more and more interconnected methods—from historic analytics to ML mannequin coaching and generative AI purposes,” mentioned Swami Sivasubramanian, vp of Knowledge and AI at AWS.
“To help these workloads, many shoppers already use combos of our purpose-built analytics and ML instruments, resembling Amazon SageMaker—the de facto commonplace for working with knowledge and constructing ML fashions—Amazon EMR, Amazon Redshift, Amazon S3 knowledge lakes, and AWS Glue.
“The following technology of SageMaker brings collectively these capabilities—together with some thrilling new options—to offer clients all of the instruments they want for knowledge processing, SQL analytics, ML mannequin growth and coaching, and generative AI, straight inside SageMaker.”
The improve contains the SageMaker Unified Studio which gives a single knowledge and AI growth setting the place customers can discover and entry the entire knowledge of their group. This software integrates key instruments from AWS, resembling Amazon Bedrock, making it simpler for customers to handle their knowledge, develop ML fashions, and construct GenAI purposes.
AWS shared that NatWestGroup, a number one financial institution group within the UK, is about to make use of SageMaker Unified Studio throughout the group to help varied workloads, together with knowledge engineering and SQL analytics. AWS claims that this unified setting will assist the financial institution cut back the time knowledge customers spend accessing analytics and AI capabilities by 50%.
As a part of its ongoing efforts to boost AI governance and enterprise safety, AWS launched the Catalog function in SageMaker. This software permits customers to outline and implement constant entry insurance policies with granular controls. Constructed on Azure Datazone, Sagemaker Catalog helps safeguard AI fashions with toxicity detection, accountable AI insurance policies, knowledge classification, and guardrails.
A key improve to the platform is the introduction of the brand new SageMaker Lakehouse. It helps cut back knowledge silos by enabling AI, ML, and analytical instruments to question and analyze knowledge throughout varied storage programs all through the group. Moreover, the platform is appropriate with Apache Iceberg open requirements, permitting clients to work with their knowledge effectively for SQL analytics.
AWS shared that Roche, a Swiss prescription drugs and diagnostics firm, anticipates a 40% discount in knowledge processing time utilizing SageMaker Lakehouse to unify knowledge from Redshift and Amazon S3 knowledge lakes. This permits companies to focus extra on reaching their strategic objectives and fewer on knowledge administration. Clients additionally get to make use of their most popular analytics and ML instruments on their knowledge, no matter the place the info is saved.
SageMaker Lakehouse helps Apache Iceberg, making it appropriate with varied AI, ML, and question instruments that use the open commonplace. It additionally gives zero-ETL integrations for Amazon Aurora MySQL, PostgreSQL, RDS for MySQL, and DynamoDB, in addition to in style SaaS purposes like Zendesk and SAP.
These integrations permit companies to effectively entry and analyze knowledge with out constructing advanced knowledge pipelines. This displays AWS’s broader technique to simplify knowledge workflows for analytics and ML, making a unified setting for knowledge processing and perception technology.
“Organizations of all sizes and throughout industries, together with Infosys, Intuit, and Woolworths, are already benefiting from AWS zero-ETL integrations to shortly and simply join and analyze knowledge with out constructing and managing knowledge pipelines,” AWS famous in a press launch.
“With the zero-ETL integrations for SaaS purposes, for instance, on-line actual property platform Idealista will be capable of simplify their knowledge extraction and ingestion processes, eliminating the necessity for a number of pipelines to entry knowledge saved in third-party SaaS purposes and releasing their knowledge engineering staff to concentrate on deriving actionable insights from knowledge somewhat than constructing and managing infrastructure.”
SageMaker’s next-generation platform is already obtainable, with the SageMaker Unified Studio at the moment in preview. Whereas AWS has not offered a particular timeline, it talked about that the SageMaker Unified Studio is anticipated to be usually obtainable quickly.
Associated Objects
AWS Bolsters GenAI Capabilities in SageMaker, Bedrock
AWS Takes On Google Spanner with Atomic Clock-Powered Distributed DBs
AWS Unveils Hosted Apache Iceberg Service on S3, New Metadata Administration Layer