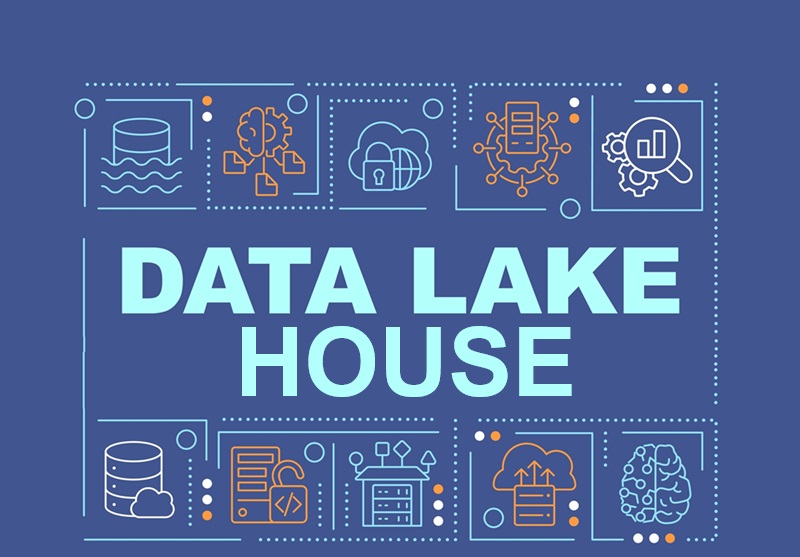
(bsd-studio/Shutterstock)
The standard information lakehouse emerged about eight years in the past as organizations sought a center floor between the anything-goes messiness of knowledge lakes and the locked-down fussiness of knowledge warehouses. The architectural sample attracted some followers, however the progress wasn’t spectacular. Nonetheless, as we kick off 2025, the info lakehouse is poised to develop fairly robustly, because of a confluence of things.
As the massive information period dawned again in 2010, Hadoop was the most well liked know-how round, because it offered a strategy to construct giant clusters of cheap industry-standard X86 servers to retailer and course of petabytes of knowledge way more cheaply than the expensive information warehouses and home equipment constructed on specialised {hardware} that got here earlier than them.
By permitting clients to dump giant quantities of semi-structured and unstructured information right into a distributed file system, Hadoop clusters garnered them the nickname “information lakes.” Clients might course of and remodel the info for his or her specific analytical wants on-demand, or what’s known as a “construction on learn” strategy.
This was fairly totally different than the “construction on write” strategy used with the everyday information warehouse of the day. Earlier than Hadoop, clients would take the time to rework and clear their transactional information earlier than loading it into the info warehouse. This was clearly extra time-consuming and dearer, however it was needed to maximise the usage of expensive storage and compute sources.
Because the Hadoop experiment progressed, many purchasers found that their information lakes had changed into information swamps. Whereas dumping uncooked information into HDFS or S3 radically elevated the quantity of knowledge they might retain, it got here at the price of decrease high quality information. Particularly, Hadoop lacked the controls that allowed clients to successfully handle their information, which led to decrease belief in Hadoop analytics.
By the mid-2010s, a number of impartial groups had been engaged on an answer. The primary workforce was led by Vinoth Chandar, an engineer at Uber, who wanted to resolve the fast-moving file drawback for the ride-sharing app. Chandar led the event of a desk format that may enable Hadoop to course of information extra like a conventional database. He known as it Hudi, which stood for Hadoop upserts, deletes, and incrementals. Uber deployed Hudi in 2016.
A yr later, two different groups launched related options for HDFS and S3 information lakes. Netflix engineer Ryan Blue and Apple engineer Daniel Weeks labored collectively to create a desk format known as Iceberg that sought to deliver ACID-like transaction capabilities and rollbacks to Apache Hive tables. The identical yr, Databricks launched Delta Lake, which melded the info construction capabilities of knowledge warehouses with its cloud information lake to deliver a “good, higher, greatest” to information administration and information high quality.
These three desk codecs largely drove the expansion of knowledge lakehouses, as they allowed conventional database information administration methods to be utilized as a layer on high of Hadoop and S3-style information lakes. This gave clients one of the best of each worlds: The scalability and affordability of knowledge lakes and the info high quality and reliability of knowledge warehouses.
Different information platforms started adopting one of many desk codecs, together with AWS, Google Cloud, and Snowflake. Iceberg, which turned a top-level Apache challenge in 2020, garnered a lot of its traction from the open supply Hadoop ecosystem. Databricks, which initially stored shut tabs on Delta Lake and its underlying desk format earlier than regularly opening up, additionally turned well-liked because the San Francisco-based firm quickly added clients. Hudi, which turned a top-level Apache challenge in 2019, was the third most-popular format.
The battle between Apache Iceberg and Delta Lake for desk format dominance was at a stalemate. Then in June of 2024, Snowflake bolstered its assist for Iceberg by launching a metadata catalog for Iceberg known as Polaris (now Apache Polaris). A day later, Databricks responded by saying the acquisition of Tabular, the Iceberg firm based by Blue, Weeks, and former Netflix engineer Jason Reid, for between $1 billion and $2 billion.
Databricks executives introduced that Iceberg and Delta Lake codecs can be introduced collectively over time. “We’re going to prepared the ground with information compatibility so that you’re not restricted by which lakehouse format your information is in,” the executives, led by CEO Ali Ghodsi, stated.
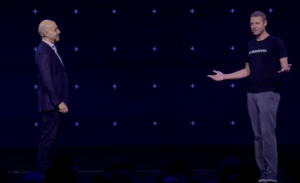
Tabular CEO Ryan Blue (proper) and Databricks CEO Ali Ghodsi on the stage at Information + AI Summit in June, 2024
The influence of the Polaris launch and Tabular acquisitions had been big, notably for the neighborhood of distributors growing impartial question engines, and it instantly drove an uptick in momentum behind Apache Iceberg. “If you happen to’re within the Iceberg neighborhood, that is go time when it comes to getting into the following period,” Learn Maloney, Dremio’s chief advertising and marketing officer, instructed this publication final June.
Seven months later, that momentum continues to be going sturdy. Final week, Dremio revealed a brand new report, titled “State of the Information Lakehouse within the AI Period,” which discovered rising assist for information lakehouses (which are actually thought-about to be Iceberg primarily based, by default).
“Our evaluation reveals that information lakehouses have reached a vital adoption threshold, with 55% of organizations operating the vast majority of their analytics on these platforms,” Dremio stated in its report, which is predicated on a fourth-quarter survey of 563 information decision-makers by McKnight Consulting Group. “This determine is projected to achieve 67% throughout the subsequent three years in keeping with respondents, indicating a transparent shift in enterprise information technique.”
Dremio says that value effectivity stays the first driver behind the expansion in information lakehouse, cited by 19% of respondents, adopted by unified information entry and enhanced ease of use (17% respectively) and self service analytics (13%). Dremio discovered that 41% of lakehouse customers have migrated from cloud information warehouses and 23% have transitioned from commonplace information lakes.
Higher, extra open information analytics is excessive on the listing of causes to maneuver to an information lakehouse, however Dremio discovered a stunning variety of clients utilizing their information lakehouse to again one other use case: AI growth.
The corporate discovered an astounding 85% of lakehouse customers are at present utilizing their warehouse to develop AI fashions, with one other 11% stating within the survey that they deliberate to. That leaves a surprising 4% of lakehouse clients saying they haven’t any plans to assist AI growth; it’s principally everybody.
Whereas AI aspirations are common at this level, there are nonetheless huge hurdles to beat earlier than organizations can truly obtain the AI dream. In its survey, Dremio discovered organizations reported critical challenges to reaching success with AI information prep. Particularly, 36% of respondents say governance and safety for AI use circumstances is the highest problem, adopted by excessive value and complexity (cited by 33%) and a scarcity of a unified AI-ready infrastructure (20%).
The lakehouse structure is a key ingredient for creating information merchandise which might be well-governed and extensively accessible, that are vital for enabling organizations to extra simply develop AI apps, stated James Rowland-Jones (JRJ), Dremio’s vp of product administration.
“It’s how they share [the data] and what comes with it,” JRJ instructed BigDATAwire on the re:Invent convention final month. “How is that enriched. How do how do you perceive it and purpose over it as an finish consumer? Do you get a statistical pattern of the info? Are you able to get a really feel for what that information is? Has it been documented? Is it ruled? Is there a glossary? Is the glossary reusable throughout views so individuals aren’t duplicating all of that effort?”
Dremio is probably greatest identified for growing an open question engine, out there beneath an Apache 2 license, that may run towards a wide range of totally different backends, together with databases, HDFS, S3, and different file programs and object shops. However the firm has been placing extra effort recently into constructing a full lakehouse platform that may run anyplace, together with on main clouds, on-prem, and in hybrid deployments. The corporate was an early backer of Iceberg with Challenge Nessie, its metadata catalog. In 2025, the corporate plans to place extra give attention to bolstering information governance, safety, and constructing information merchandise, firm executives stated at re:Invent.
The largest beneficiary of the rise of open, Iceberg-based lakehouse platforms are enterprises, who’re not beholden to monolithic cloud platforms distributors that need to lock clients’ information in to allow them to extract more cash from them. A aspect impact of the rise of lakehouses is that distributors like Dremio now have the flexibility to promote their wares to clients, who’re free to select and select a question engine to satisfy their particular wants.
“The information structure panorama is at a pivotal level the place the calls for of AI and superior analytics are reworking conventional approaches to information administration,” Maloney stated in a press launch. “This report underscores how and why companies are leveraging information lakehouses to drive innovation whereas addressing vital challenges like value effectivity, governance, and AI readiness.”
Associated Objects:
How Apache Iceberg Received the Open Desk Wars
It’s Go Time for Open Information Lakehouses
Databricks Nabs Iceberg-Maker Tabular to Spawn Desk Uniformity