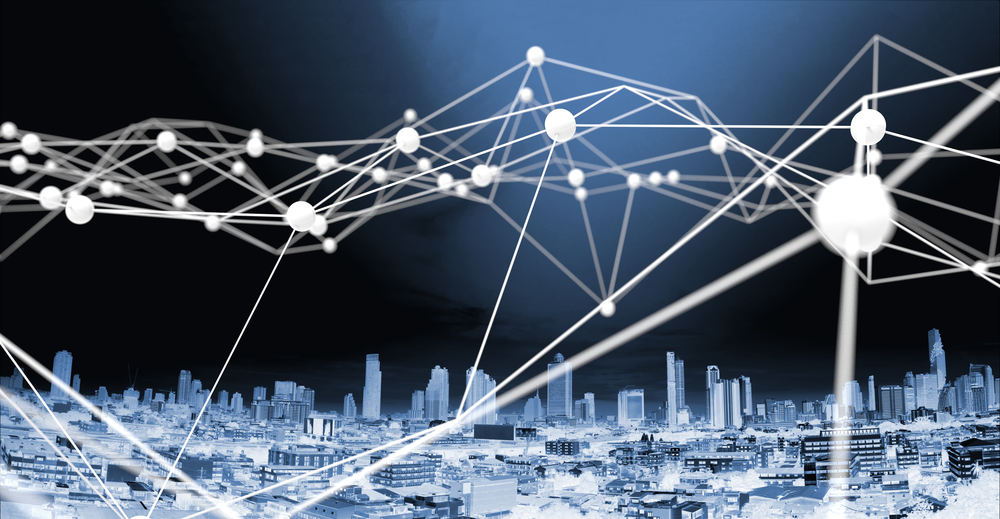
(Zapp2Photo/Shutterstock)
We’ve all heard the expression that knowledge is the lifeblood of recent organizations, however it’s actually an enterprise’s capacity to grasp its knowledge that’s invaluable. Data graphs give enterprises a deep understanding of their knowledge by performing as a collective “widespread sense” for the group. They do that by deriving insights from the relationships and context that exist between knowledge. This enhanced understanding empowers enterprises to make extra knowledgeable, constant choices that drive optimistic enterprise outcomes.
Now, enter retrieval-augmented era (RAG). In easy phrases, RAG is a course of that optimizes the output of huge language fashions (LLMs) so they supply extra correct, dependable info. When RAG is enhanced with data graphs (often known as GraphRAG), it considerably improves the accuracy and long-term reasoning skills of LLMs.
GraphRAG remains to be in its infancy, however there’s good purpose to consider it might improve LLM accuracy by as much as 3 times, in accordance with a current paper. GraphRAG is poised to usher within the subsequent period of generative AI, and can ultimately lead us to neuro-symbolic AI, the “Holy Grail” of AI know-how.
Let’s take a more in-depth take a look at the unimaginable potential of this know-how pairing.
Addressing RAG’s Limitations with Data Graphs
Data graphs tackle the restrictions related to RAG in two key methods.
First, they add extra construction to uncooked textual content knowledge by linking items of knowledge that exist inside totally different paperwork. Second, data graphs use a greater search technique to retrieve essentially the most related info. This improves LLM accuracy and reduces the possibility of hallucinations occurring.
The evolution of GraphRAG might be likened to the transition from AltaVista, one of many first internet search engines like google and yahoo, to Google. AltaVista performed internet retrieval based mostly on key phrases alone, which was helpful, however solely marginally so. Google utterly revolutionized search when it retrieved outcomes based mostly on each key phrases and PageRank, which took into consideration the significance and relevance of every webpage in relation to the key phrase searched. That is basically what GraphRAG is doing: traversing a graph of knowledge and utilizing context to supply essentially the most related, correct solutions.
Answering Extremely Advanced Questions with GraphRAG
GraphRAG can reply extremely advanced, summary questions on issues that, at first, might appear to have little to no connection to the untrained eye. Listed below are a number of examples:
Q: Which two airways can be cousins in Greek mythology?
A: Helios and Atlas.
No single piece of documentation exists to reply this query, i.e. the reply can’t be discovered on Google or in a e-book. As a substitute, GraphRAG should join the dots between disparate knowledge sources to purpose the reply. It first identifies which airways are named after figures in Greek mythology, after which examines each Helios’ and Atlas’ household bushes to substantiate their relation to 1 one other.
Q: How do Microsoft’s gross sales impression the variety of malaria instances in Rwanda?
A: As Microsoft’s gross sales enhance, malaria instances in Rwanda lower over time.
Once more, there isn’t any particular documentation that explicitly solutions this query. GraphRAG makes the connection that, when Microsoft gross sales enhance, the Invoice & Melinda Gates Basis invests more cash into malaria analysis and remedy, which in flip reduces instances of the illness in Rwanda.
Utilizing GraphRAG to Overcome Enterprise Challenges
Whereas the earlier examples are fairly summary as an instance GraphRAG’s unimaginable reasoning capabilities, the instance under illustrates a extra believable state of affairs a enterprise would possibly encounter when asking its LLM provide chain questions.
A house enchancment firm worries that fires in Arizona would possibly have an effect on their operations. They ask the questions:
- What are well-liked gadgets which might be low in stock that ship from Arizona?
- If some gadgets that come from Arizona exit of inventory what different merchandise are affected?
Whereas info concerning every of those elements (distributors, gross sales, instruments, stock, delivery location, and many others.) exists someplace, these knowledge sources usually are not linked and extremely tough to trace down manually. Due to this fact, answering these seemingly easy provide chain questions requires GraphRAG to uncover essentially the most correct, well timed solutions that take every issue—and their relation to 1 one other—into consideration.
Trying Ahead: Key Advantages and Concerns for GraphRAG
As famous, GraphRAG’s key profit is its exceptional capacity to enhance LLMs’ accuracy and long-term reasoning capabilities. That is essential as a result of extra correct LLMs can automate more and more advanced and nuanced duties and supply insights that gas higher decision-making.
Moreover, higher-performing LLMs might be utilized to a broader vary of use instances, together with these inside delicate industries that require a really excessive stage of accuracy, equivalent to healthcare and finance. That being stated, human oversight is important as GraphRAG progresses. It’s very important that every reply or piece of knowledge the know-how produces is verifiable, and its reasoning might be traced again manually by way of the graph if crucial.
In as we speak’s world, success hinges on an enterprise’s capacity to grasp and correctly leverage its knowledge. However most organizations are swimming in tons of of 1000’s of tables of knowledge with little perception into what’s really happening. This may result in poor decision-making and technical debt if not addressed.
Data graphs are crucial for serving to enterprises make sense of their knowledge, and when mixed with RAG, the chances are infinite. GraphRAG is propelling the subsequent wave of generative AI, and organizations who perceive this might be on the forefront of innovation.
Concerning the creator: Nikolaos Vasiloglou is the VP of Analysis for ML at RelationalAI, the place he leads analysis and strategic initiatives on the intersection of Massive Language Fashions and Data Graphs. He has spent his profession constructing ML software program and main knowledge science initiatives in retail, internet advertising and safety. He’s additionally a member of the ICLR/ICML/NeurIPS/UAI/MLconf/KGC/IEEE S&P neighborhood, having served as an creator, reviewer and organizer of Workshops and the primary convention.